Program
Day 1: June 21, 2023 | ||||
Welcome 8:45-8:50 EDT | ||||
8:50-9:00 Dr. Moe Khaleel, Associate Laboratory Director, National Security Sciences Directorate, Oak Ridge National Laboratory | ||||
Session 1 Moderators - Dr. Carter Christopher, Distinguished R&D Staff Member and Section Head, Oak Ridge National Laboratory and Dr. Dawn King, Senior AI/ML Geophysical Earth Scientist, National Geospatial-Intelligence Agency | ||||
A decade of evolution in sensing instruments and computing hardware is behind the astounding rise of AI and its impact on society. AI technology is now at the frontline of diagnosing diseases, translating languages, and transcribing speech. Yet in the context of geographic knowledge discovery, a growing number of emerging national security and open science challenges are revealing glaring weaknesses in current GeoAI systems. In this 3rd in a series of workshops, we seek to understand both existing and emerging grand geospatial use cases and the limiting factors to advancing the well-being and security of our society. | ||||
Key Questions |
| |||
Panelists |
| |||
10:30–11:00 Networking Break | ||||
Session 2 Moderators - Dr. Dalton Lunga, GeoAI Group Lead & Sr. R&D Scientist, Oak Ridge National Laboratory and Dr. Rahul Ramachandran, Senior Research Scientist, National Aeronautics and Space Administration | ||||
AI-enabled analytic tools promise to synthesize available Earth observation data more effectively and provide unexpected, impactful solutions relevant to various national security and climate science challenges, such as energy grid resilience, nuclear nonproliferation, and risk-based predictive systems for complex socio-economic and physical environments. But currently, the development of application-specific GeoAI models is resource intensive and costly in gathering large amounts of high-quality data, relearning common representations, and underutilization of other unlabeled data modalities. This puts the community at a significant disadvantage in advancing cutting-edge AI-enabled geospatial workflows to maintain decision superiority for national security and open science challenges. Join us in this forward-looking session to discuss the identification and production of relevant foundation GeoAI models for decision support. Our charge is to probe the emerging trends in large AI models and formulate building blocks pertinent to the trillion-pixel GeoAI challenges emerging from natural and man-made events. | ||||
Key Questions |
| |||
Panelists |
| |||
12:30-14:00 Lunch | ||||
Session 3 14:00–15:30 Geospatial Data Infrastructure Moderators - Dr. Yan Liu, Computational Scientist, Oak Ridge National Laboratory and Dr. Manil Maskey, Senior Research Scientist, National Aeronautics and Space Administration | ||||
Massive geospatial datasets collected from surveys, sensing, and social media provide rich data sources and geospatial context for GeoAI. These datasets are then curated to fit the needs of a specific application. However, the GeoAI community still faces enormous challenges integrating large, spatially heterogeneous, multi-modal geospatial datasets into existing machine learning frameworks. This session will discuss major geospatial data challenges and opportunities related to Analysis Ready Data (ARD) and interoperability of multimodal data products, including difficulties in labeling massive geospatial datasets, the development of geospatial data schemas for machine learning models, data curation and validation, the potential to leverage existing rich data sources for GeoAI, HPC-based data-intensive AI computations, and, consequently, future needs for geospatial data infrastructure solutions that couple geospatial processes, data analytics, and AI as a scalable platform for empowering large-scale applications. | ||||
Key Questions |
| |||
Panelists |
| |||
15:30–16:00 Networking Break | ||||
Session 4 16:00–17:00 Edge Computing Moderators - Professor Katie Schuman, University of Tennessee and Dr. David Page, Distinguished R&D Staff Member and Section Head, Oak Ridge National Laboratory | ||||
Geospatial edge devices — which include satellites, drones, and other systems that are typically size, weight, and power limited — play an important role in the emergence of trillion pixel challenges. These edge devices are the front line of the emerging global geospatial enterprise that collects daily more than 100 trillion pixels over the surface of the Earth. The current trend of connecting these edge systems to large, federated “cloud” computing demands ever-increasing bandwidth to handle the volume and velocity of raw data where a single satellite generates more than 100 terabytes per day. The primary mode of connection is through wireless networks to push this volume of data from the edge to centralized processing. Unlike this high-bandwidth network-based approach, edge computing offers an opportunity to reduce bandwidth requirements and to improve latency and security whereby processing and computing are distributed into the edge devices and condensing raw data into more manageable information. GeoAI, neuromorphic computing, quantum computing, and other advances offer exciting research avenues for edge computing to address SWAP limitations while simultaneously reducing downstream bandwidth requirements and enhancing decision-making loops. At this session, we will discuss the state of edge computing, identify enabling technologies and grand challenges, and define future research directions to make edge computing a disrupting agent for GeoAI-based decision-making. | ||||
Key Questions |
| |||
Panelists |
| |||
17:30 Dinner on Campus | Keynote: Better (geospatial) data, greater (societal) relevance: challenges and opportunities on the path to a net-zero world, Dr. David McCollum, Sr. R&D Staff, Oak Ridge National Laboratory | |||
Day 2 June 22, 2023 | ||||
Session 5 09:00–10:30 HPC Hardware and Software Architectures Moderators - Professor Eric Shook, University of Minnesota and Dr. Jitendra Kumar, Research Staff Member, Oak Ridge National Laboratory | ||||
Application of GeoAI to growing volumes of geospatial information will require efficient, high-performance computing (HPC) hardware and network infrastructure and scalable software frameworks to transfer, store and process massive amounts of data. Planetary-scale GeoAI architectures capable of learning and inferring from trillions of pixels streaming daily will require tight integration of network, storage, and computing resources that span CPUs, GPUs, accelerators, and potentially domain-specific architectures such as a GeoAI spatial processor. Also needed are scalable open-source software ecosystems to analyze these datasets leveraging state-of-the-art computational resources. This session will explore the computational challenges facing GeoAI and discuss potential and holistic architectural solutions to overcome these challenges. | ||||
Key Questions |
| |||
Panelists |
| |||
10:30-11:00 Networking Break | ||||
Session 6 11:00–12:00 Workforce Requirements and Partnerships Moderators - Professor Shawn Newsam, University of California, Merced and Dr. Lexie Yang, Research Scientist, Oak Ridge National Laboratory | ||||
Effective partnerships will be fundamental for addressing the challenges and opportunities arising in the multimodal GeoAI frontier. Researchers and practitioners with expertise in different GeoAI data modalities will need to come together to make progress on societal-scale challenges. Enabling AI model-based solutions to solve GeoAI grand challenges will require even more interdisciplinary collaboration due to the scale of resources and complexities of policy-making needed to sustain the ecosystem. What types of talent do we need to bring together now to build such an ecosystem? What types of talent do we need to train to sustain and extend it? What are the key elements needed to foster a workplace or collaborative environment that will support generations to carry on the mission and solve the grand challenges? | ||||
Key Questions |
| |||
Panelists |
| |||
12:00 Closing Remarks. Followed by a Guided Tour of Frontier Supercomputer (the World's Fastest) and Summit Supercomputer ( the World's 5th Fastest) |
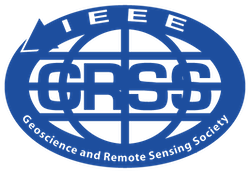